Machine Learning Helps Diagnose Specific MD Forms, Study Finds
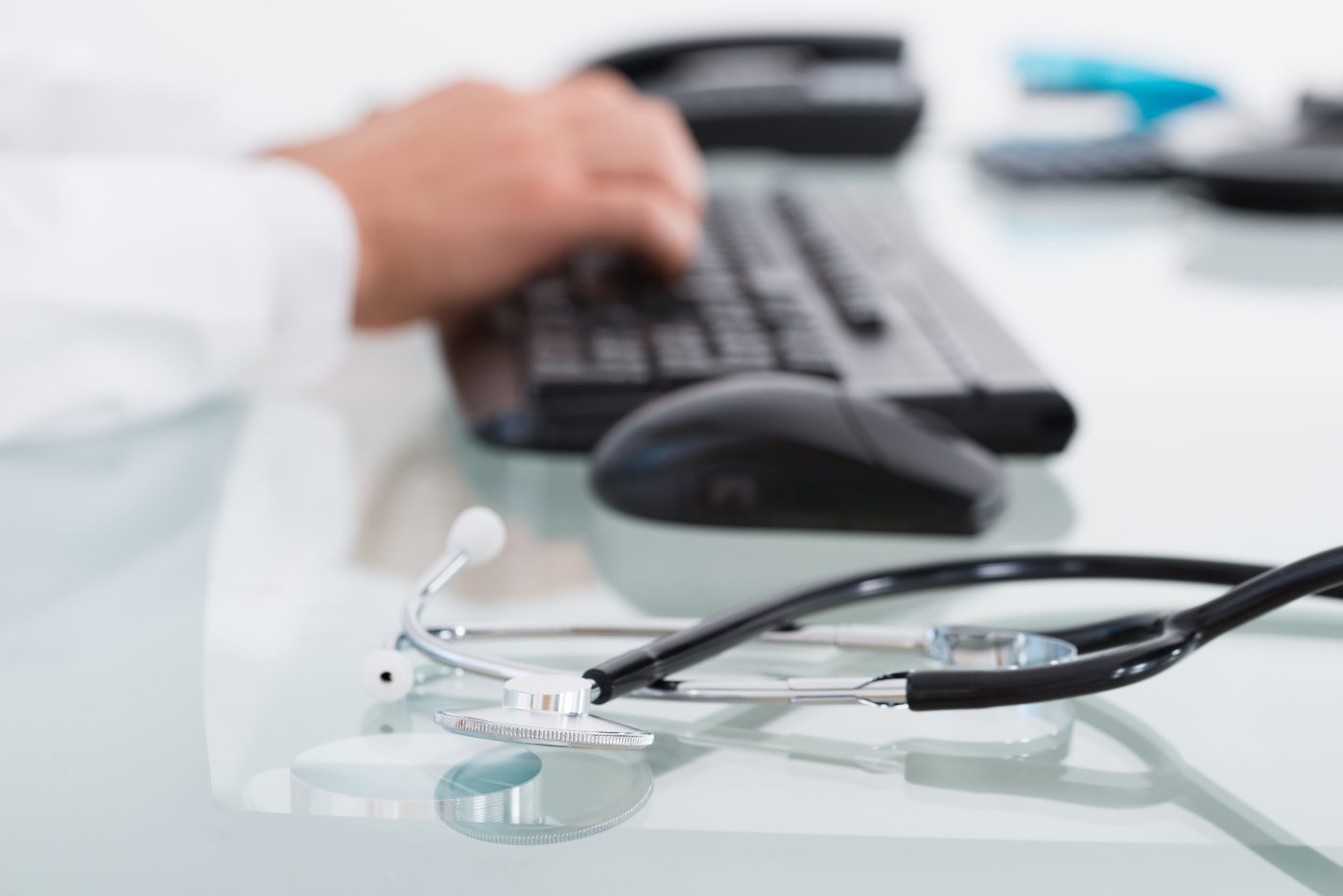
Machine learning could be used to help diagnose specific forms of muscular dystrophy (MD) using magnetic resonance imaging (MRI) scans, a study suggests.
The study, “Accuracy of a machine learning muscle MRI-based tool for the diagnosis of muscular dystrophies,” was published in the journal Neurology.
MD diagnosis generally relies on genetic testing to identify mutations. This is supplemented with clinical findings, including MRI of affected muscles. In the case of MD, MRI most commonly identifies places where muscle tissue is replaced by fat tissue. However, the overlap between different MDs in terms of how they look on an MRI scan requires a high degree of specialization to distinguish specific disease forms.
Researchers from Spain used machine learning to develop a tool to aid in the diagnosis of MDs based on MRI data.
The team collected 986 pelvis and leg muscle MRIs of people with genetically confirmed diagnosis of MDs, including Duchenne and Becker MD (46 scans combined), as well as multiple types of limb-girdle MD (LGMD). Ten scans were excluded because they did not show fat replacement.
The scientists used a machine learning strategy to find a model capable of differentiating the disorders. They split the data into training and validation groups.
The model provided a set of potential diagnoses for every case, ordered from most probable to least probable, with the investigators considering an answer correct if the first proposed diagnosis was the correct one.
The final model had a diagnostic accuracy of 95.7%, with an overall sensitivity (correct diagnosis of MD subsets) of 92.1%, and a specificity (accurate exclusion of an MD type) of 99.4%.
The researchers also looked at the model’s ability to differentiate between conditions with similar muscle involvement, such as certain subsets of LGMD. Results showed an accuracy of greater than 90%.
The strategy was then compared to diagnosis made by experts in the field, who analyzed 20 MRIs of confirmed MD cases and were asked to propose a first, second, and third diagnosis. These were converted into scores: experts received three points if their first diagnosis was correct, two points if their second was correct, and one if the third was correct, for a possible total of 60 points among all 20 images.
While the four experts scored within 42 and 31 points, the machine learning algorithm outperformed them all, scoring 55 points.
The researchers are not proposing the model as the sole approach for diagnosis, which needs confirmation by genetic testing. However, it could point to particular avenues for further diagnostic tests, and the researchers are planning to make it available for free online.
“This tool can be of great help in the diagnostic process of MDs, providing potential genes to be tested or reinforcing the pathogenic role of a mutation found,” they said. “This study can be considered as a proof of concept that demonstrates that artificial intelligence can be applied to the field of muscle MRI.”